The Importance of Data Quality for Asset Management
Industrial assets are crucial parts of any industrial facility. While most industrial assets are designed and built to last a long time, under operating conditions, wear and tear occurs. Careful inspection and maintenance procedures are crucial to detect wear and tear when it occurs and make repairs when and where necessary. That way, industrial assets can be protected from costly failures.
While it is widely known and accepted that inspection and maintenance are crucial aspects of asset management, Data Quality is observed to be a somewhat neglected part of these procedures. In the absence of Quality Data, effectiveness of inspection and maintenance may be significantly reduced. Inspection results are reached by processing obtained data and where and when maintenance is to be conducted is based on such results. Thus, if the data is shaky, the whole practice of asset management is affected.
Low quality data, even when it may be used to ensure compliance, does more harm than good. Low quality data may cause inspection to be ineffectual, since false results may incorrectly predict failure when asset is healthy or vice versa. Thus, low quality data can endanger asset integrity, cause OHS and/or environmental risks and incur unnecessary costs on the asset owner. Low quality data may be caused by various factors such as faulty or obsolete equipment, inexperienced operators or difficult conditions.
Getting quality data is ensured by working with quality equipment, experienced personnel and using cutting edge methods utilizing the latest technology. AIS Field’s main purpose is obtaining high quality and abundant data for industrial assets in a quick and safe manner. In this post, we go over the important aspects of the concepts of Data Quality and Quality Data and how AIS Field can help you in obtaining high quality data.
What is Quality Data?
Quality data can be defined as information that is accurate, reliable, consistent, and fit for its intended use. It is critical for making informed decisions. Quality Data can be defined as having several qualities.
First of all, it is accurate. Accuracy means being reflective of real-life condition. No data can be 100% accurate, but it should be as accurate as possible. Quality Data has as little deviation as possible and thus it is gathered with the method that is proven to be the most accurate.
Secondly, Quality Data is complete, in the sense that it contains all the data points necessary. Even the most accurate data is not Quality Data if it lacks information on some of the most important points.
Thirdly, it is consistent and repeatable. If a measurement is not repeatable, ie. it changes from measurement to measurement, it cannot be deemed reliable.
Fourthly, it should be timely. In operating conditions, using old data may be very costly. These are the most crucial qualities of Quality Data.
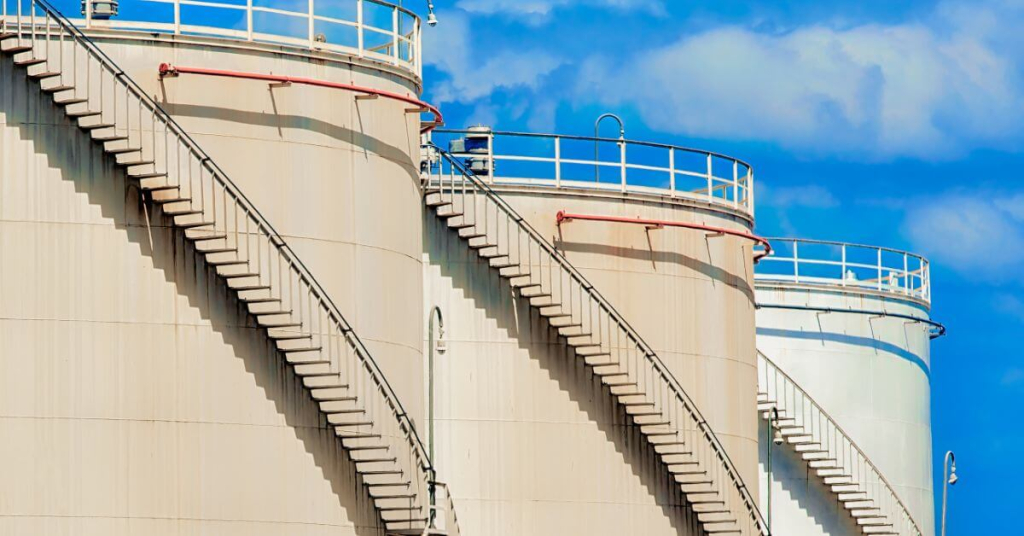
The Importance of Data Quality
Industrial asset management includes maintenance and upkeep procedures and lifecycle management. Neither of these can be efficient in the absence of Quality Data. Maintenance activities, such as preventive, predictive, and corrective maintenance, depend on Quality Data to assess the condition of assets, predict failures, and schedule repairs.
Without accurate and timely data on asset performance, maintenance teams cannot identify early signs of failure and conduct predictive maintenance. Predictive maintenance itself is based on the availability of Quality Data. If data is not available or Data Quality is low, false negatives or false positives may occur, leading to either unexpected failure or unnecessary maintenance, both of which are unwanted outcomes. If data is known to be reliable, asset lifecycle can be more easily managed, and maintenance schedules may be more accurately set. That way, assets can be kept in operation in the safest and least costly manner for the longest time.
The Cost of Poor Data Quality
Nowadays, the importance of data collection in industrial asset management is well understood. Periodic NDT is also regulated by the authorities and thus mandatory. However, not every data is created equal. NDT data on the assets are based on the quality of the equipment as well as the experience and skill of the NDT operator. When data collected does not have the features needed to be deemed Quality Data, it is poor quality data. Poor quality data can still be useful and may even be mostly correct enough, however, the most important feature of poor quality data is that it is not reliable.
Poor quality data may cause false positives for defects, in the sense that data indicates non-existent defects. This phenomenon may lead to unnecessary maintenance to be conducted, meaning costs and downtime. On the other hand and maybe even worse, poor quality data may fail to detect an existing defect (false negative). When that happens, maintenance can be skipped when it is indeed needed, leading to unexpected failure. Most of the time asset owners are aware that data quality is low, thus they do not fully depend on inspection data and conduct more maintenance than indicated by the data. The extra cost of these extra maintenance sessions adds up to a great number during an asset’s lifetime.
How to Gather Quality Data?
As mentioned before, Quality Data requires quality operation. Ensuring data accuracy is only made possible by using the method proper for the operation. In addition to that, the device should be functioning correctly, should be correctly calibrated and should be used properly.
Ensuring data completeness is another aspect of gathering quality data. For a dataset to be complete, data gathering should have been made from many different locations. Sometimes these locations require working at heights or working in confined spaces.
In short, data completeness can be costly and sometimes dangerous. It requires more man-hours and additional equipment. Consistency and repeatability in data is another issue that can be overcome by experienced operators and good inspection practices. However, human operators are not machines; thus, they will always have a certain level of inconsistency. As we will see in the next section, AIS Field’s robotic solutions make all these significantly easier to achieve and ensure quality data.
How AIS Field can Help You?
AIS Field’s RUVI Oildiver and RUVI Wallker robots are automated robotic solutions for gathering data safely, quickly and accurately from industrial assets. When applicable, robotic NDT has numerous benefits over traditional NDT practices. Robots are configured to reach the accuracy needed for the NDT operation that is to be conducted.
Our robots provide fully repeatable and consistent data, which is impossible for human operators. In addition, robotic NDT gathers tens of thousands time more data than that is possible for human operators. This data can be gathered without any safety precautions, any scaffolding, or, in the case of RUVI Oildiver, even in-service. To ensure Data Quality and obtain Quality Data, robotic NDT is the next step forward.
Robotic NDT data is not presented as specific points, but a full mapping of the inspected asset. That way, robotic NDT provides reliable and thorough data and makes it possible to base efficient maintenance planning upon it.